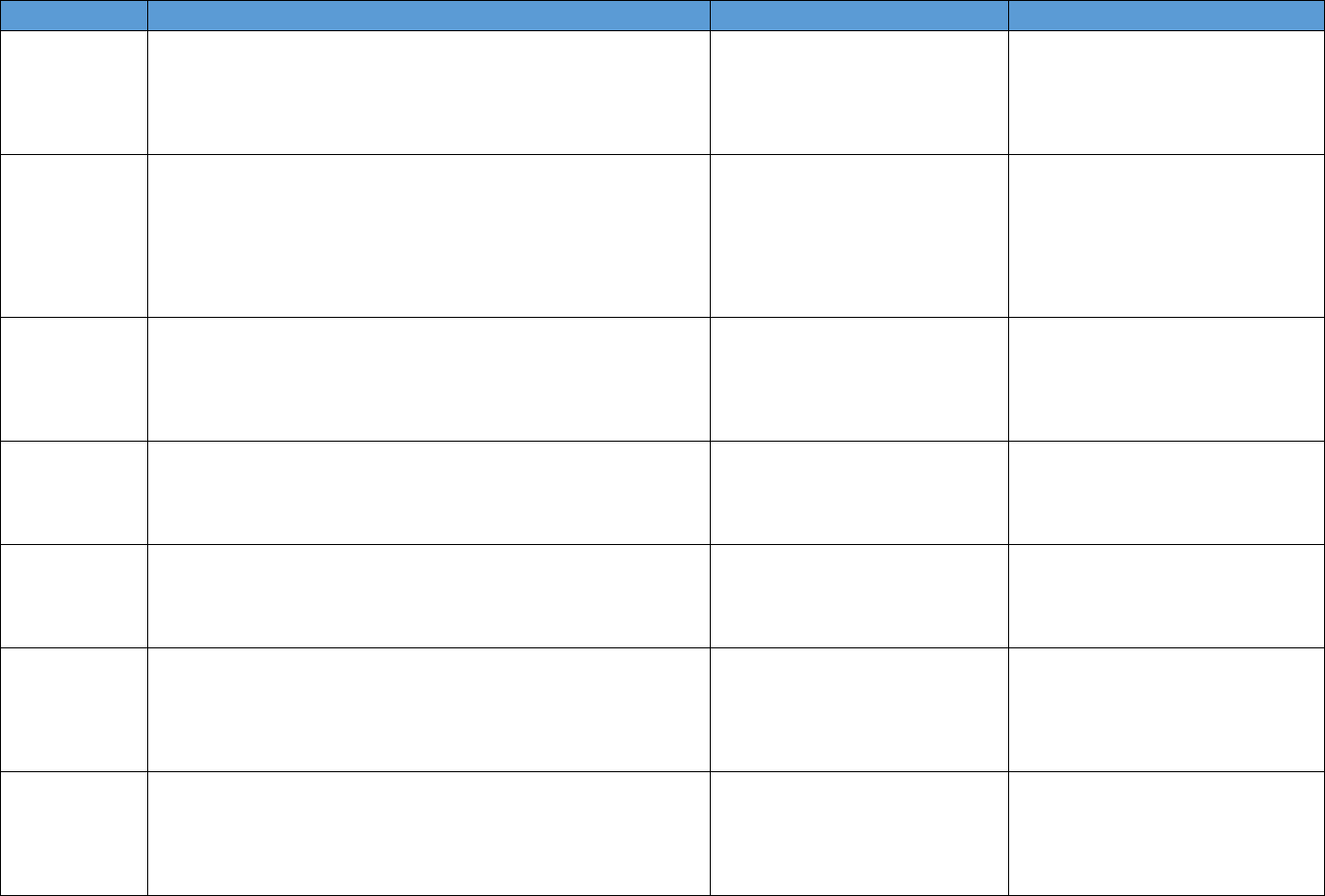
Table 4.1. Data Source Categories Identified
Data Description Summary Examples of Important Measures Data Source Examples
National surveys
Description: Generally household or school4based surveys with self4
reported information on drug use and health; other surveys are of
hospitals, treatment facilities, or other medical service providers
Geographic coverage: National
Timing: Generally collected and available annually
Prescription opioid use, heroin use,
opioid use disorder, medical
conditions, health care utilization
National Survey on Drug Use and
Health, National Ambulatory Medical
Care Survey, National Survey of
Substance Abuse Treatment Services
Data, Medical Expenditure Panel
Survey
EHR Description: An EHR contains the medical and treatment histories of
patients. However, it often contains more than standard clinical data,
and may also include a broader view of a patient’s care. EHRs may
contain a
patient’s medical history, diagnoses, medications, treatment
plans, allergies, radiology images, and laboratory and test results
Geographic coverage: Varies by source
Timing: Near4real time or real4time collection
Previously prescribed opioids or
other medications; patient history,
medications, clinical conditions,
treatment plans, and lab/test
results; may include clinician notes
Stanford Translational Research
Integrated Database, HealthCore
Integrated Research Database, Group
Health Cooperative in Washington
State
Claims data
Description: Patient4level claims data for reimbursement for services
submitted by health care providers and pharmacies to insurance
companies. Validated algorithms to identify opioid misuse or abuse
from claims data are being developed
Geographic coverage: Varies by source
Timing: Varies by source
Prescription drug utilization; service
utilization
IQVIA, Symphony Health, Truven
Marketscan data, Medicaid claims,
Medicare Part D Prescription Drug
Event data
Mortality records
Description: Death rates and causes of death by drug compound
and/or International Classification of Diseases code. Additional
information can include toxicology reports
Geographic coverage: National or single state
Timing: Generally available annually
Rates of opioid4involved deaths;
drugs involved in overdose deaths
CDC WONDER Multiple4cause4of death
data; Fatal Accident Reporting System;
NDI
Prescription
monitoring data
Description: Data systems to track and monitor the distribution or
prescription of controlled substances
Geographic coverage: Varies by source
Timing: Varies by source
Opioid prescribing rates (by type);
indicators of "doctor shopping,"
coprescribing of opioids and other
controlled drugs, geographic
variation in opioid distribution
Automation of Reports and
Consolidated Orders System (ARCOS);
state prescription drug–monitoring
programs
Contextual and
policy data
Description: Causal analyses of the effects of policy changes on
opioid4related outcomes generally use data on state laws from these
sources and/or includes controls for state or county characteristics to
support causal interpretation
Geographic coverage: National
Timing: Varies, but generally semiannually
State opioid policies, state and
county demographic and
socioeconomic factors, state and
county health care variables
Area Health Resources Files, Policy
Surveillance System, PDAPS
Other national,
state, and local
sources
Description: Includes data collected through law enforcement,
national public health surveillance systems (e.g., poison control center
data, emergency department visit data), OEND program data, other
hospitalization and emergency department data
Geographic coverage: Varies by source
Timing: Varies by source
Law enforcement drug seizures,
nonfatal opioid overdose, opioid4
related emergency department visits
and hospitalizations, naloxone
distribution through community
organizations
NEMSIS, NPDS, HCUP emergency
department and hospitalization data
18